Introduction
Eubacterial organisms have genomes that vary largely in their nucleotide compositions. In this kingdom, the GC content varies from 20% to 70% of the genome and this large variation has been documented in a number of reports that have aimed to explain it1–3. The amino acid compositions are also different in eubacterial proteomes due to the variation of GC content4. It has been reported that these difference of amino acid compositions alter the characteristics of proteomes and as a consequence, proteins of GC-poor genomes are more prone to misfolding and aggregation compare to GC-rich genomes5,6. It has been hypothesized that GroEL plays a major role, if not an essential role, in the evolution of GC-poor organisms by buffering deleterious mutations that are fixed due to population bottlenecks7–9. This has been supported by the observation that many of the small GC-poor endosymbionts tend to overexpress GroEL10–12.
However, the proposed chaperone dependence of GC-poor organisms does not explain why some of the GC-poor endosymbionts of the mycoplasma group have lost the groEL copy from their genome13. It is notable that these are the only known eubacterial organisms to have lost this gene. This observation led us to test the proposed relationship of GC poorness of genome with the aggregation propensity of the encoded proteome.
Obtaining information on the aggregation propensity of proteins from different organisms is a challenging task. However, there has already been a careful characterization of the aggregation propensity of different Escherichia coli proteins that was conducted in a high-throughput manner14–16. Kerner et al. classified the GroEL substrates into Class I, II or III based on the interaction strength and on the stringency of their requirement for GroEL. Class III (C3) substrates were completely dependent on GroEL for folding, whereas Class II (C2) substrates were partially dependent. Class I (C1) proteins interacted weakly with GroEL and were able to fold spontaneously. In a trivial approach, homologs of GroEL-dependent proteins may be identified in other organisms13,17. This approach however fails to predict the evolution of protein dependence on GroEL correctly, as the sequence differences between species have the potential to introduce or remove kinetic traps from folding pathways, thereby altering their dependence on GroEL. In addition to the solubility of the E. coli proteome in a chaperone-free system, substrates of another chaperone DnaK were also identified by two independent research groups18,19. Applications developed primarily on machine learning algorithms to classify soluble or GroEL substrates16,18,20–24 are already available. However, these classifiers have not been trained with curated data prepared from multiple experimental results14,15,18,19. In this study, we have constructed a more reliable training dataset to build classifiers to determine the aggregation propensity and GroEL dependency in 1132 eubacterial proteomes, based solely on the amino acid sequences. We show a distinct trend in the aggregation propensity of proteins of an organism in relation to the GC content. Surprisingly, aggregation propensity decreased with lower GC content independent of symbiotic characteristics, suggesting that GC-poor organisms have indeed evolved a proteome that is devoid of aggregation-prone proteins.
Materials and methods
Data source
The aggregation-prone proteins of the eSOL database18,25 are dependent on the chaperone network of E. coli to get their three dimensional native structure. GroEL and DnaK are two important components of this network and their substrates have been extensively studied via different experimental methods14,15,19,26. The integration of all the available information reveals that about half (457) of the soluble or chaperone-independent proteins identified by Niwa et al. were found to be GroEL- or DnaK-dependent18 (Figure 1). To construct a more reliable training set, we removed these proteins from the soluble set. Thus, proteins identified as chaperone-dependent by more than one study, were only considered as aggregation-prone proteins. Furthermore, the proteins which were more than 30% (amino acid) sequence similarity among the remaining proteins were removed using CD-HIT27 clustering program. Therefore the final training set comprised of 502 aggregation prone and 475 soluble proteins.
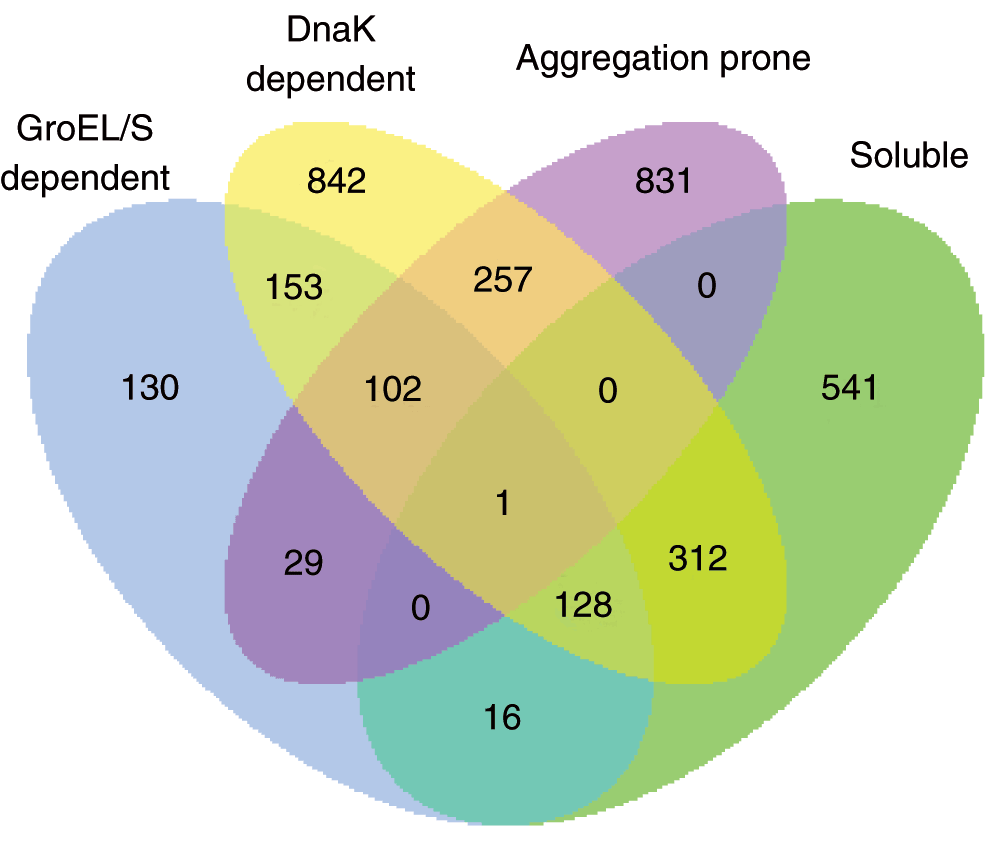
Figure 1. Integration of independent studies.
A Venn diagram of proteins of E. coli identified by different experimental studies shows that ~45% of soluble proteins reported by Niwa et al. overlap with GroEL/S or DnaK substrates (soluble proteins are defined as having solubility >70% and aggregation-prone proteins have solubility <30%).
Classifier building
The classifiers in this study were built with Pro-Gyan28 software. Pro-Gyan builds classifiers directly from training data set given in FASTA format by selecting relevant features from a large number of unbiased features. Following metrics which are useful to evaluate performance of machine learning classifiers were reported by Pro-Gyan.
Accuracy(Acc)= (TP + TN)/(TP + TN + FP + FN)
Sensitivity or Recall (Sn) = TP/(TP + FN)
Sencificity (Sp) = TN/(FP + TN)
Matthews correlation coefficient (MCC) = (TP*TN–FP*FN)/
where TP = True Positive, TN = True Negative, FP = False Positive, FN = False Negative predicted by the classifier.
Additionally, receiver operating characteristic (ROC) curves and area under this curve (AUC)29 were also generated.
Analysis on microbial genomes
The protein sequences of microbial genomes were downloaded from the Microbial Genome Database30 (archive no. mbgd_2011-01). To identify the chaperonins in the microbial organisms, chaperonin homologs were searched for using BLAST (e-value 1*10-4) against a chaperonin database cpnDB31 downloaded on June 2011. The 16S rRNA nucleotide sequence of E. coli was acquired from SILVA32 and homologous were searched for in other microbial organisms using BLAST (e-value 1*10-4). GC contents for microbial genomes were calculated using following equation
GC content = (G + C)/(total bases),
where G = number of guanosine and C = number of cytosine.
Statistical analysis
The Kendall correlation and analysis of covariance were performed in R33 statistical computing environment using the package ‘stats’ version 2.15.3. To account the effect of evolution on different traits of bacterial genomes, we performed phylogenetic independent contrast through the PDAP34 module on Mesquite35 application.
Results and discussion
Development of machine learning tool to identify aggregation-prone proteins
Recently protein solubility has been carefully measured in a chaperone-free system and the information has been made available through the eSol database18. Few classification models developed on this database can segregate soluble proteins from chaperone-dependent proteins22–24. However, these web-based classifiers are not suitable to classify large numbers of proteomes, and their soluble or negative training dataset (proteins not aggregation-prone or soluble) are not carefully curated, as most of the soluble proteins from eSol database are substrates of DnaK19 or GroEL14,15 (Figure 1). Therefore we built a classifier containing a curated list of aggregation-prone proteins and soluble proteins. The classifier was built using Pro-Gyan28 which generates 5038 different features from a set of class labelled protein sequences and selects the “maximum relevant minimum redundant” feature subset. Finally, the tool built a support vector machine (SVM)36 classifier by five-fold cross validation. The classifier attained an accuracy of 83.21% with 0.66 MCC (Table 1). Although Pro-Gyan generated classifier was trained with a rigorously curated training data set, it performs equivalent to Fang et al.’s classifier and better than others22–24. The receiver operating characteristic (ROC) curves of the classifier are shown in Figure 2. For interested users, the classifier is available in ZENODO (https://zenodo.org/record/10442).
Table 1. Comparison of previous classifiers with our classifier.
Method | Sensitivity | Specificity | Accuracy | AUC | MCC |
---|
SVM25 | | | 80 | | |
J48 (decision tree algorithm)23 | | | 72 | 0.72 | |
VTJ48 (visually tuned J48)23 | | | 76 | 0.81 | |
Fang et al.22 | 82.00 | 85.00 | 84 | 0.91 | 0.67 |
SolubEcoli.pgc*
|
86.25
|
80.00
|
83.21
|
0.88
|
0.66
|
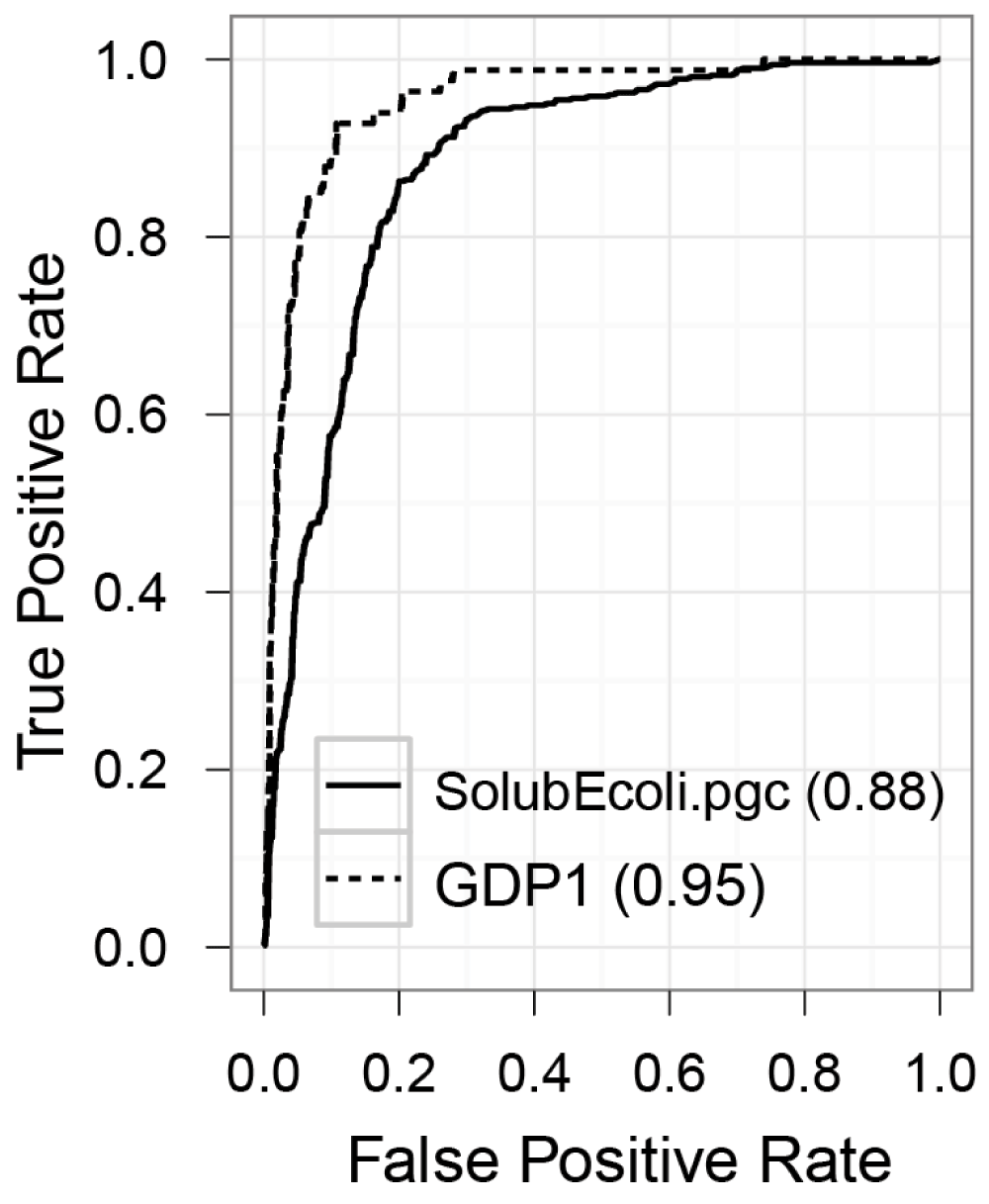
Figure 2. Receiver operating characteristic (ROC) curves.
ROC curves of the soluble protein classifier (SolubEcoli.pgc) and the GroEL obligate protein classifier (GDP1.pgc). The areas under the curves (AUC) are given in the legend.
Discriminating features of aggregation prone proteins
To build the classifier, Pro-Gyan28 selected 24 relevant features through an automated process. The top ten significant (by Mann-Whitney test) features were the sequence patterns, the pseudo amino acid composition37 of phenylalanine (F), aspartic (D) and glutamic (E) acid, the distribution of positively charged amino acids, the features calculated from FoldIndex38 and the auto-correlation of hydrophobicity and relative mutability (Table 2). The remaining selected features (Table 2) were enriched with auto-correlation measurement of amino acid indices such as steric parameter, free energy, accessible surface area, polarizability, residue volume etc. The features which represent patterns of physico-chemical properties encrypted in protein sequences were unique to SolubEcoli.pgc when compared to earlier methods.
Table 2. Selected features of proteins used to build the “SolubEcoli.pgc” classifier.
Serial no. | Feature id† | Description | p-value* |
---|
1 | SW_SOC2 | Quasi-sequence-order calculated from physicochemical distance matrix50. | 2.20E-16 |
2 | PPR | Distribution of positively charged amino acids in sequence pattern51. | 2.20E-16 |
3 | H(8)M | Amino acid pair composition of histidine to methionine with 8 gaps52. | 2.33E-15 |
4 | M-B(Hydr)1 | Moreau-Broto auto correlation (lag 1) of amino acid index; hydrophobicity53. | 2.24E-08 |
5 | PseAAC_T1_3 | Pseudo amino acid composition of aspartic acid (D)37. | 9.45E-06 |
6 | PseAAC_T1_5 | Pseudo amino acid composition of phenylalanine acid (F)37. | 6.87E-05 |
7 | FI_16_psavgl | Average length of folded segments of proteins according to FoldIndex38. | 8.14E-05 |
8 | PseAAC_T1_4 | Pseudo amino acid composition of glutamic acid (E)37. | 0.000542 |
9 | Dstrbu_Pol_2:3 | Distribution of amino acids according to polarizability54. | 0.001289 |
10 | M-B(mutblty)6 | Moreau-Broto auto correlation (lag 6) of amino acid index; relative mutability53. | 3.65E-03 |
11 | T | Composition of amino acid Threonine53. | 5.00E-03 |
12 | Mrn(vlum)27 | Moran auto correlation (lag 27) of amino acid index; residue volume53. | 0.00926 |
13 | Mrn(Polar)22 | Moran auto correlation (lag 22) of amino acid index; polarizability53. | 0.013 |
14 | M-B(mutblty)9 | Moreau-Broto auto correlation (lag 9) of amino acid index; relative mutability53. | 0.01988 |
15 | Geary(sterc)4 | Geary auto correlation (lag 4) of amino acid index; steric parameter53. | 0.03536 |
16 | M-B(mutblty)24 | Moreau-Broto auto correlation (lag 24) of amino acid index; relative mutability53. | 0.05416 |
17 | M-B(Hydr)12 | Moreau-Broto auto correlation (lag 12) of amino acid index; hydrophobicity53. | 5.92E-02 |
18 | Mrn(RsdAcc)24 | Moran auto correlation (lag 24) of amino acid index; residue accessible surface area in tripeptide53. | 0.1077 |
19 | Mrn(Hydr)23 | Moran auto correlation (lag 23) of amino acid index; hydrophobicity53. | 0.2106 |
20 | Geary(Free)13 | Geary auto correlation (lag 13) of amino acid index; free energy53. | 0.3271 |
21 | Comp_Vol_2 | Composition of normalized van der Waals volume of amino acids of range 2.95–4.053. | 0.4631 |
22 | Geary(vlum)20 | Geary auto correlation (lag 20) of amino acid index; residue volume53. | 4.95E-01 |
23 | Geary(Free)14 | Geary auto correlation (lag 14) of amino acid index; free energy53. | 0.499 |
24 | M-B(vlum)30 | Moreau-Broto auto correlation (lag 30) of amino acid index; residue volume53. | 0.9559 |
Genome wide prediction of aggregation prone proteins
From the analysis of features, it was noticed that the compositions of amino acids are significantly different within aggregation prone and soluble proteins. Sequence features of amino acids have been used to understand protein overexpression related to toxicity39. Additionally, it has been also shown that the amino acid composition is drastically altered in organisms with GC-poor genomes4,40. There are multiple amino acids that change in frequency as a function of GC content (Figure 3) and this change that has been attributed to the difference in the GC content in the codons of these amino acids. On the basis of these differences, it has been reported that proteins encoded by GC-poor organisms should be more prone to aggregation than proteins encoded by GC-rich organisms5,6. However, the GC composition of the training data showed that aggregation-prone proteins were significantly more GC-rich than the soluble proteins (Figure 4, Mann-Whitney test p-value = 1.3e-15). Subsequently, we sought to verify the fraction of aggregation-prone proteins across different bacterial proteomes. We used the SolubEcoli.pgc classifier to predict aggregation-prone proteins in 1132 eubacterial species. Our prediction on bacterial genomes showed that the fAg (aggregation prone proteins as fraction of proteome) of a genome correlates positively with the GC composition (Kendall tau=0.38 p-value < 2.2e-16) (Figure 5A). We further examined the correlation, with respect to phylogenetic ancestry, using the Mesquite system35, because the Kendall correlation assumes that observations are independent even if organisms are linked through common ancestors41. The required phylogenetic tree was constructed from the 16S rRNA gene sequences of 570 bacteria42. We found a significant correlation (0.4, p-value < 2.2e-16) between independent contrasts of GC content and fAG (Figure 5B). This corroborated well with the difference seen between soluble and aggregation-prone proteins in E. coli (Figure 4). Thus the increase in the GC composition of a genome may encode proteome that harbours a higher fraction of aggregation-prone proteins.
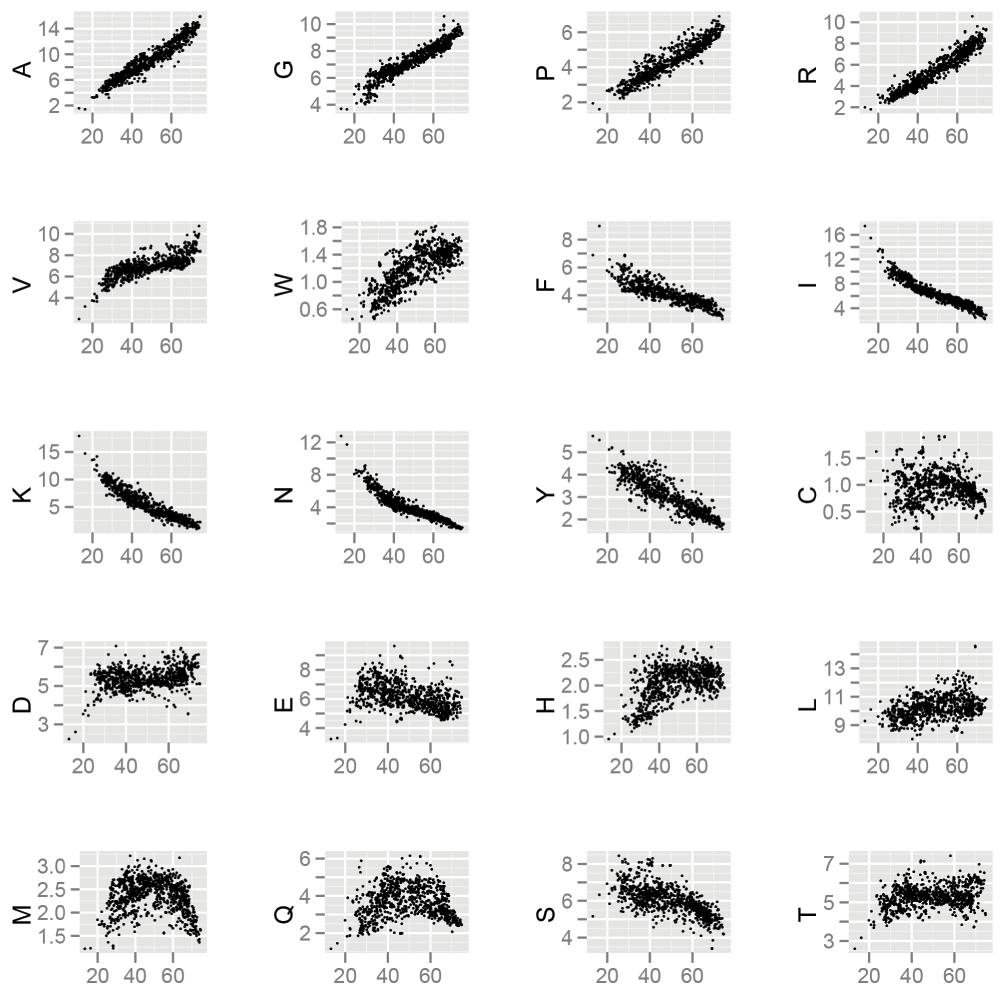
Figure 3. Composition of basic amino acids over ~1100 eubacterial genomes.
The x-axis of each subplot shows for GC composition of each genome whereas y-axis shows corresponding amino acid composition.
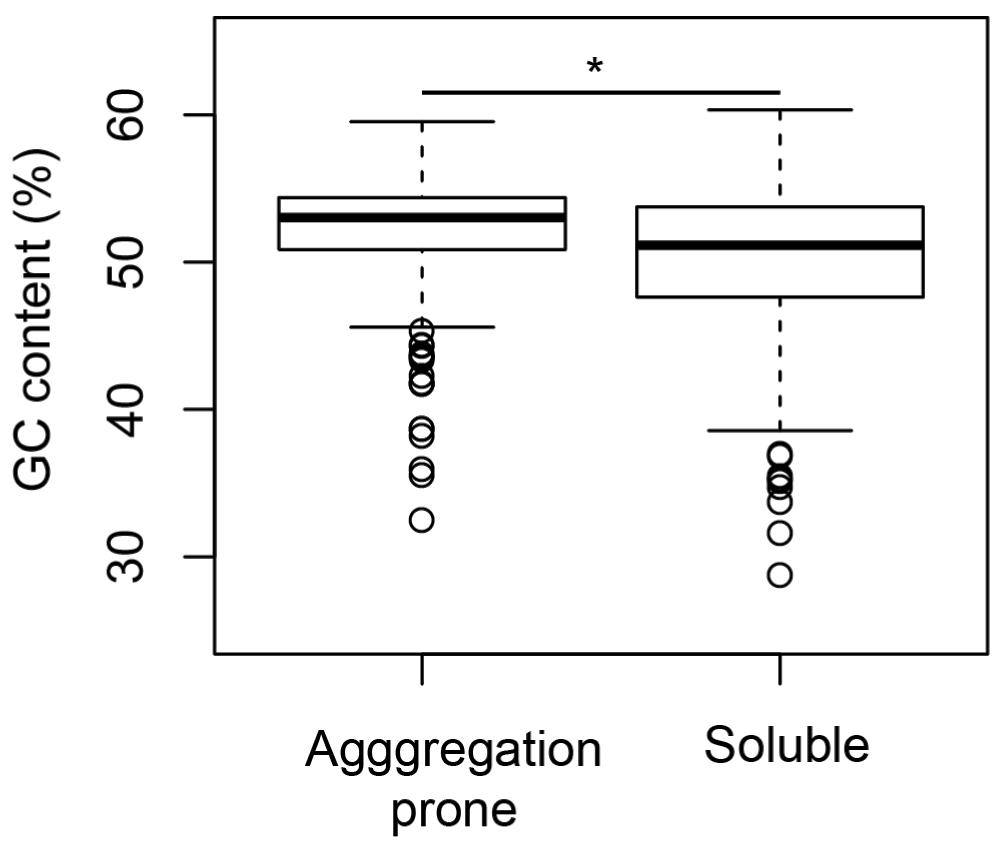
Figure 4. Aggregation-prone proteins are richer in GC-content than soluble proteins.
In E. coli, aggregation-prone proteins contain higher GC-content than soluble proteins. Mann-Whitney test p-value (*) is 1.3e-15.
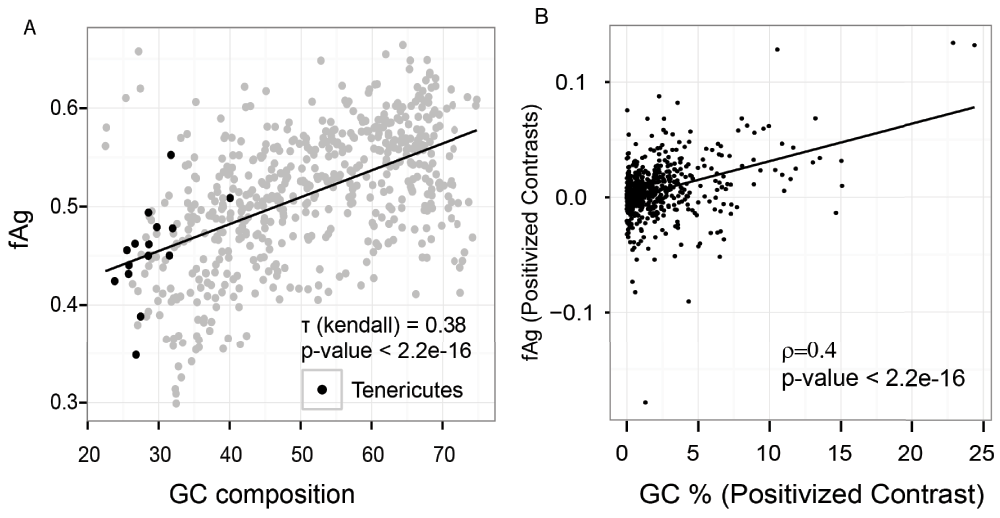
Figure 5. GC content is associated with fAg.
(A) GC content of the genome correlates with the fraction of proteome that is aggregation-prone (fAg) (analysis of 570 bacterial genomes using the classifier). Rank-based correlation is provided along with the p-value. The black line shows a linear regression model. (B) The relationship between GC content and fAg was obtained through a phylogenetically independent contrast method (570 bacteria). A positive correlation (0.4) was identified between GC content and fAg (p-value < 2.1e-16).
This is in contrast to previous reports hypothesizing that GC-poor organisms have unstable and aggregation prone proteomes. Notably, the earlier hypothesis that GC poorness is associated with GroEL-dependent aggregation-prone proteomes was based on the observation that GroEL is overexpressed in GC-poor organisms. Therefore, to segregate GroEL-dependent proteins from aggregation-prone proteomes, we developed another classifier (ZENODO, https://zenodo.org/record/10442/) trained with 475 curated soluble and 83 GroEL obligate (Class 3 or C3) proteins14. The classifier achieved an accuracy of 92.29% with MCC of 0.69. We used GDP1.pgc to identify the C3 proteins within aggregation-prone proteins (predicted by SolubEcoli.pgc) to examine the evolution of the GroEL-dependent proteome with GC composition. Indeed we found that the fC3 (fraction of C3 proteins) of bacterial proteome are more correlated with GC content than the fAg fraction (Figure 6A). The phylogenetically independent contrasts of fC3 and GC also correlated strongly (0.7, p-value < 2.2e-16, Figure 6B). The phylum Tenericutes, members of which have GC-poor genomes, was predicted to encode less GroEL-dependent proteins. Mycoplasma and Ureaplasma are the main genera of the phylum Tenericutes and many species of these groups lack GroEL43. In our analysis, we also observed that the Tenericutes without GroEL (red dots in Figure 6A) had very few fC3 proteins. This motivated us to investigate the effect of groEL copy number on misfolded proteins. Interestingly, there was a strong correlation between the groEL copy number and the fraction of genome coding for C3 proteins (Figure 6C). Due to the presence of noise in the experimental data, we tried to benchmark the classifiers. Fujiwara et al. reported that five C3 homologs of groEL-lacking Ureaplasma urealyticum are soluble in GroEL depleted cells26. Hence, we also examined the tolerance of our classifiers by predicting the GroEL dependency of these homologs. Four of these homologs were predicted to be GroEL independent with a high confidence score (Table 3). Overall, the results indicated that C3 proteins and in general aggregation-prone proteins do decrease with the GC content of genomes.
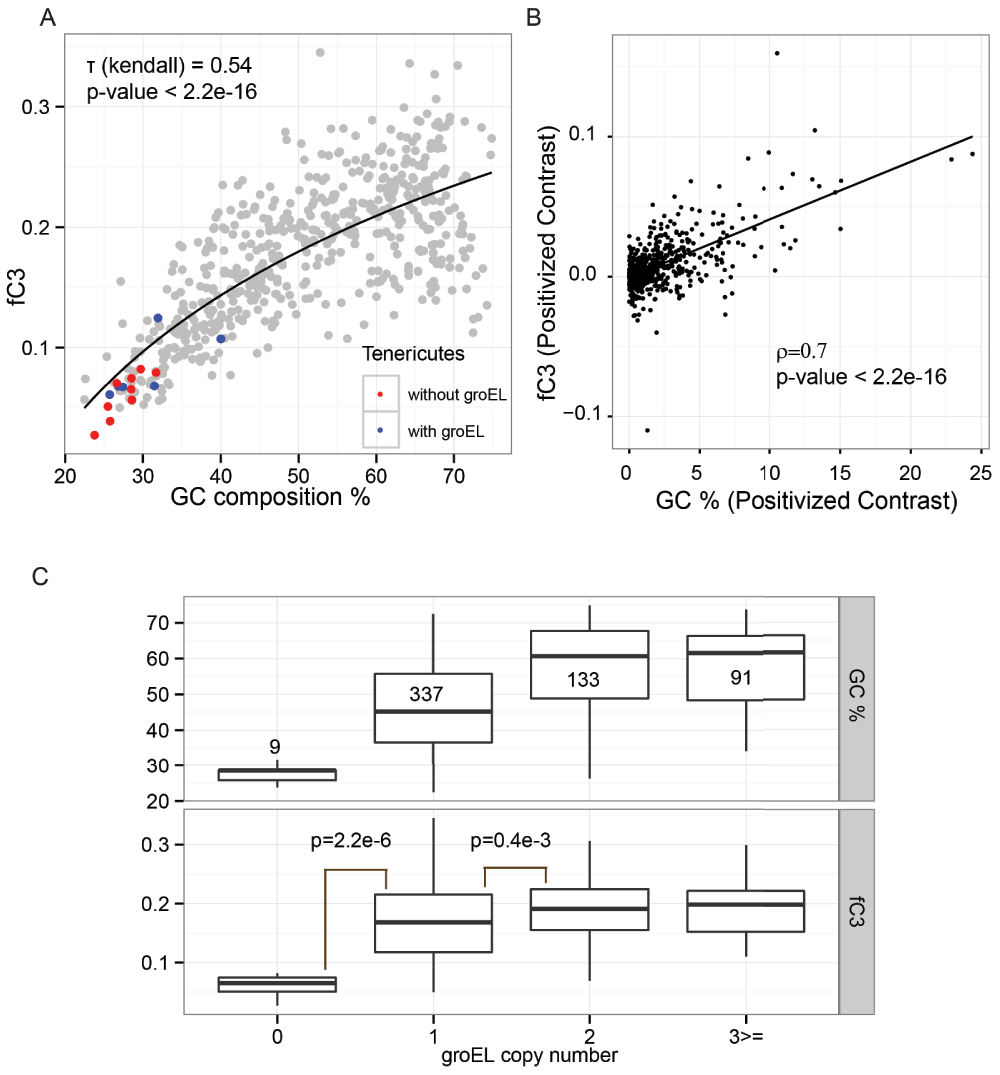
Figure 6. Decrease in GC content is associated with decrease in fC3.
(A) Correlation of GC content with the fraction of the proteome that is GroEL obligate (fC3) over 570 bacterial genomes. Members of the phylum Tenericutes with and without the groEL gene are coloured in blue and red, respectively. Rank-based correlation is provided along with p-value. The black line shows a logarithmic regression model. (B) A positive correlation (0.7) was identified between independent contrast of GC content and fC3 with respect to phylogenetic information of bacterial genomes (570 bacteria, p-value < 2.2e-16). (C) The organisms were classified based on the number of groEL genes present in the genome. fC3 exhibited a significant increase with an increase in the number of genome-encoded groEL copies. The p-values were calculated by Mann-Whitney test using two-sided hypothesis.
Table 3. Evaluation of classifiers on five C3 homologous proteins of groEL-lacking Ureaplasma urealyticum.
The homologous were found in U. urealyticum by NCBI BLAST at a threshold of E value of 1e45. Then the aggregation propensity and GroEL dependency of these proteins were classified by SolubEcoli.pgc and GDP1.pgc.
C3 homologous proteins in Ureaplasma urealyticum | E value | Accession | Is aggregation prone? (classifier: SolubEcoli.pgc) | Is GroEL dependent? (classifier: GDP1.pgc) |
---|
UuMetK | 2e-99 | YP_002284849.1 | Yes (0.739) | No (0.804) |
UuDeoA | 2e-80 | WP_004026878.1 | Yes (0.586) | No (0.938) |
UuCsdB | 4e-62 | D82890 | Yes (0.884) | Yes (0.665) |
UuGatY | 8e-46 | H82870 | Yes (0.672) | No (0.973) |
UuYcfH | 7e-41 | E82944 | Yes (0.518) | No (0.881) |
Correlation of GC content with protein solubility is independent of the population bottleneck
Endosymbionts are crucial to this study as the literature suggests that these organisms have undergone bottlenecks during evolution44. It is hypothesized that these organisms have accumulated more deleterious mutations compared to non-endosymbionts8. If this were true then endosymbionts should show a greater aggregation propensity or dependence on GroEL than that predicted by the GC content of free-living eubacterial species. To measure the impact of a symbiotic relationship on C3 proteins, we performed an analysis of covariance ANCOVA on 570 eubacterial species42. There was no significant effect of a symbiotic relationship on fAG/fC3 (p-value 0.24/0.65, Data set) or significant interaction (p-value=0.36/0.38) with GC composition (Figure 7). Thus we were unable to obtain proof for any association of a bottleneck in evolutionary history with protein aggregation propensity. Therefore we rule out the possibility of bottleneck evolution as the reason for the evolution of GroEL-independent proteomes like Ureaplasma and GroEL-independent mycoplasma species.
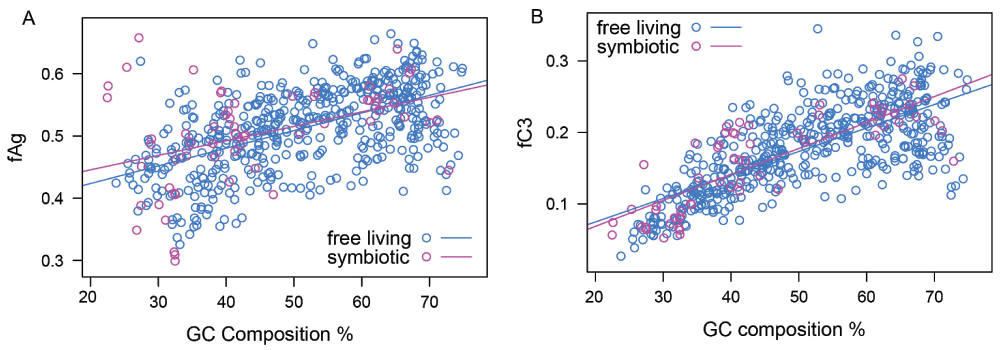
Figure 7. fAG and fC3 are correlated to the GC content independent from the species habitat.
The ANCOVA test on 570 organisms showed that a symbiotic relationship has no significant effect or interaction with GC content on the aggregation propensity or GroEL-dependency of the proteins of an organism.
Name | Genome Size (MB) | GC Content | GroEL copy number | fC3 | fAg |
---|
A.arabaticum | 3.205686 | 61.892088 | 1 | 0.26 | 0.56 |
A.aeolicus | 1.590791 | 43.30160279 | 1 | 0.21 | 0.55 |
A.ferrooxidans_DSM10331 | 3.918192 | 59.27175085 | 3 | 0.16 | 0.48 |
A.ferrooxidans_ATCC23270 | 2.313035 | 42.23096494 | 1 | 0.16 | 0.47 |
A.fermentans | 2.469596 | 36.63129516 | 1 | 0.07 | 0.32 |
A.asiaticus | 1.884364 | 35.04503376 | 1 | 0.18 | 0.53 |
A.ferrooxidans | 2.204665 | 44.28568513 | 1 | 0.14 | 0.45 |
A.cellulolyticus | 5.226648 | 62.42985944 | 2 | 0.15 | 0.52 |
A.phosphatis | 5.352772 | 68.53230438 | 1 | 0.27 | 0.59 |
A.bacterium | 5.650368 | 58.38384332 | 1 | 0.22 | 0.59 |
A.baumannii_AB307-0294 | 3.760981 | 39.04369631 | 1 | 0.15 | 0.50 |
A.baumannii_ACICU | 3.996761 | 38.93301351 | 1 | 0.15 | 0.49 |
A.baumannii_SDF | 3.477996 | 39.11962521 | 1 | 0.23 | 0.54 |
A.baumannii_AB0057 | 4.059242 | 39.19490388 | 1 | 0.14 | 0.49 |
Acinetobacter_ADP1 | 3.120143 | 54.7277801 | 1 | 0.23 | 0.55 |
A.dehalogenans | 2.341251 | 27.04515663 | 1 | 0.06 | 0.41 |
A.baumannii_AYE | 4.048735 | 39.31590978 | 3 | 0.15 | 0.51 |
A.baumannii | 4.001457 | 38.92091056 | 1 | 0.13 | 0.46 |
A.vinosum | 4.152543 | 38.73207333 | 1 | 0.14 | 0.50 |
A.mirum | 2.44354 | 66.90735572 | 2 | 0.20 | 0.58 |
A.mediterranei | 4.98087 | 65.95859759 | 2 | 0.14 | 0.48 |
A.ehrlichei | 3.598621 | 40.4295701 | 1 | 0.16 | 0.52 |
A.laidlawii | 1.496992 | 31.92775913 | 1 | 0.12 | 0.48 |
A.cryptum | 1.206806 | 49.98491887 | 1 | 0.21 | 0.56 |
A.colombiense | 1.980592 | 45.30685775 | 2 | 0.21 | 0.59 |
Anaeromyxobacter_Fw109-5 | 5.029329 | 74.71648802 | 2 | 0.26 | 0.60 |
A.radiobacter | 3.96308 | 67.09526429 | 2 | 0.27 | 0.62 |
A.dehalogenans_2CP-1 | 5.013479 | 74.90527037 | 2 | 0.28 | 0.62 |
A.acidocaldarius | 2.157067 | 59.46514411 | 2 | 0.29 | 0.58 |
Acinetobacter_DR1 | 3.275944 | 67.53125206 | 1 | 0.33 | 0.57 |
A.tumefaciens | 4.308776 | 59.50808304 | 1 | 0.21 | 0.56 |
A.pleuropneumoniae | 2.885038 | 58.85049001 | 1 | 0.24 | 0.55 |
A.oremlandii | 2.846746 | 41.77675142 | 1 | 0.20 | 0.50 |
A.metalliredigens | 2.329769 | 55.84270372 | 2 | 0.23 | 0.58 |
A.haemolyticum | 2.158157 | 68.28933206 | 2 | 0.19 | 0.55 |
A.pleuropneumoniae_AP76 | 2.982397 | 58.77245719 | 1 | 0.22 | 0.51 |
A.butzleri | 5.27799 | 73.53140116 | 2 | 0.28 | 0.61 |
A.pleuropneumoniae_JL03 | 4.744448 | 61.54840352 | 4 | 0.23 | 0.58 |
A.arilaitensis | 1.986154 | 53.13324143 | 1 | 0.14 | 0.50 |
Acidovorax_JS42 | 4.585154 | 66.08755126 | 1 | 0.25 | 0.61 |
V.fischeri_MJ11 | 3.669074 | 64.1861407 | 2 | 0.28 | 0.56 |
A.vitis | 1.197687 | 49.76266754 | 1 | 0.20 | 0.56 |
A.succinogenes | 4.44898 | 44.8854007 | 1 | 0.14 | 0.46 |
A.aurescens | 10.236715 | 71.29279266 | 4 | 0.16 | 0.53 |
A.centrale | 1.202435 | 49.77449925 | 1 | 0.21 | 0.57 |
A.chlorophenolicus | 8.248144 | 73.70887317 | 3 | 0.17 | 0.50 |
A.marina | 8.361599 | 46.95589324 | 4 | 0.12 | 0.41 |
A.degensii | 4.929566 | 36.82202449 | 2 | 0.11 | 0.41 |
A.muciniphila | 2.664102 | 55.76235444 | 1 | 0.28 | 0.62 |
Cyanothece_PCC7424 | 7.211789 | 41.26833162 | 3 | 0.12 | 0.48 |
A.nitrofigilis | 5.061632 | 74.8406838 | 2 | 0.27 | 0.61 |
Anaeromyxobacter_K | 3.192235 | 28.36085063 | 1 | 0.08 | 0.43 |
A.prevotii | 3.123558 | 36.25730657 | 1 | 0.10 | 0.45 |
A.salmonicida | 2.345435 | 41.22629704 | 1 | 0.16 | 0.51 |
Azospirillum_B510 | 2.753527 | 48.85370654 | 1 | 0.15 | 0.47 |
A.marginale_Florida | 1.471282 | 41.63593383 | 1 | 0.12 | 0.40 |
A.actinomycetemcomitans | 2.242062 | 41.23284726 | 1 | 0.15 | 0.51 |
A.hydrophila | 2.274482 | 41.29951347 | 1 | 0.16 | 0.52 |
A.xylosoxidans | 5.306133 | 63.96925972 | 1 | 0.27 | 0.60 |
A.flavithermus | 1.998633 | 35.63890919 | 1 | 0.10 | 0.36 |
A.pseudotrichonymphae | 1.224919 | 32.94781124 | 1 | 0.16 | 0.60 |
A.marginale | 3.340249 | 53.09951444 | 1 | 0.24 | 0.57 |
Arthrobacter_FB24 | 1.543805 | 45.68659902 | 1 | 0.12 | 0.48 |
A.phagocytophilum | 7.2733 | 59.8742249 | 3 | 0.21 | 0.57 |
A.parvulum | 5.070478 | 65.38845056 | 3 | 0.16 | 0.52 |
A.aphrophilus | 5.040536 | 58.18095536 | 3 | 0.22 | 0.54 |
A.salmonicida_LFI1238 | 2.319663 | 44.91859378 | 1 | 0.17 | 0.56 |
B.amyloliquefaciens | 2.931662 | 35.19164215 | 2 | 0.12 | 0.50 |
A.excentricus | 5.674258 | 59.04114688 | 1 | 0.19 | 0.54 |
Cyanothece_ATCC51142 | 7.105752 | 41.40974805 | 4 | 0.13 | 0.50 |
A.caulinodans | 6.320946 | 57.47074884 | 2 | 0.21 | 0.57 |
V.fischeri | 5.365318 | 65.67554803 | 2 | 0.29 | 0.56 |
A.avenae | 7.359146 | 65.7789912 | 2 | 0.24 | 0.63 |
Phytoplasma_AYWB | 0.72397 | 26.81174634 | 1 | 0.07 | 0.35 |
A.pasteurianus | 5.369772 | 67.31902211 | 2 | 0.22 | 0.61 |
B.bacilliformis | 7.599738 | 67.61279139 | 3 | 0.25 | 0.60 |
Diaphorobacter | 4.37604 | 67.91644958 | 3 | 0.27 | 0.61 |
A.vinelandii | 0.618379 | 25.34157855 | 1 | 0.09 | 0.61 |
Azoarcus_BH72 | 7.642536 | 66.39171867 | 3 | 0.23 | 0.57 |
B.cavernae | 2.089645 | 59.18433514 | 1 | 0.18 | 0.53 |
B.cereus | 4.168266 | 43.21929071 | 1 | 0.14 | 0.40 |
B.afzelii | 1.232503 | 27.70321857 | 1 | 0.06 | 0.39 |
B.anthracis_CI | 5.506763 | 35.24665943 | 1 | 0.10 | 0.35 |
B.anthracis_Ames0581 | 5.503926 | 35.24295203 | 1 | 0.10 | 0.37 |
B.anthracis_Sterne | 5.486649 | 35.25310258 | 1 | 0.11 | 0.39 |
B.ambifaria_MC40-6 | 7.528567 | 66.77483245 | 4 | 0.23 | 0.58 |
B.anthracis_A0248 | 5.227293 | 35.37683845 | 1 | 0.10 | 0.37 |
B.anthracis | 3.980199 | 46.08234412 | 1 | 0.15 | 0.43 |
A.macleodii | 0.642122 | 26.28565911 | 1 | 0.11 | 0.61 |
B.anthracis_CDC684 | 5.503926 | 35.24295203 | 1 | 0.10 | 0.36 |
B.aphidicola_5A | 0.641454 | 25.3305771 | 1 | 0.12 | 0.60 |
B.atrophaeus | 5.228663 | 35.37944595 | 1 | 0.10 | 0.38 |
B.aphidicola_Bp | 0.641895 | 26.29246216 | 1 | 0.11 | 0.60 |
B.avium | 3.732255 | 61.58258211 | 1 | 0.23 | 0.59 |
B.amyloliquefaciens_DSM7 | 3.918589 | 46.47565744 | 1 | 0.16 | 0.44 |
B.bacteriovorus | 3.78295 | 50.64550153 | 1 | 0.19 | 0.56 |
B.cereus_03BB102 | 6.296436 | 47.27355285 | 1 | 0.15 | 0.46 |
B.animalis_lactis_DSM10140 | 2.186882 | 62.75843873 | 1 | 0.16 | 0.44 |
B.quintana | 1.445021 | 38.23868304 | 1 | 0.18 | 0.51 |
Blattabacterium_Blattella_germanica | 0.63685 | 27.14171312 | 1 | 0.16 | 0.66 |
B.bifidum | 2.214656 | 62.66643668 | 1 | 0.17 | 0.47 |
B.bronchiseptica | 5.339179 | 68.07702083 | 2 | 0.27 | 0.63 |
B.suis_ATCC23445 | 8.493513 | 64.80619974 | 6 | 0.21 | 0.55 |
B.burgdorferi | 1.519856 | 28.17066222 | 1 | 0.06 | 0.35 |
B.burgdorferi_ZS7 | 1.345494 | 28.26709001 | 1 | 0.07 | 0.42 |
B.cereus_NVH | 5.419036 | 35.30356691 | 1 | 0.10 | 0.38 |
B.floridanus | 0.422434 | 20.20007859 | 1 | 0.07 | 0.55 |
B.cereus_AH187 | 5.427083 | 35.28834919 | 1 | 0.10 | 0.38 |
B.cereus_Q1 | 5.736823 | 35.01549551 | 1 | 0.10 | 0.37 |
B.pertussis | 7.70284 | 66.78837935 | 3 | 0.23 | 0.58 |
B.licheniformis | 4.303871 | 44.75331626 | 1 | 0.15 | 0.45 |
B.petrii | 7.971389 | 66.59509403 | 3 | 0.23 | 0.58 |
B.parapertussis | 7.279116 | 66.92514586 | 2 | 0.23 | 0.58 |
B.clausii | 5.506207 | 35.46797278 | 1 | 0.10 | 0.39 |
B.cereus_G9842 | 5.599857 | 35.51051393 | 1 | 0.10 | 0.37 |
B.tribocorum | 3.312769 | 57.24265712 | 1 | 0.19 | 0.52 |
B.bifidum_PRL2010 | 4.669183 | 73.11658164 | 2 | 0.13 | 0.45 |
B.cereus_B4264 | 5.449308 | 35.33263306 | 1 | 0.10 | 0.37 |
B.cereus_ZK | 4.094159 | 35.86699979 | 1 | 0.13 | 0.42 |
B.halodurans | 5.843235 | 35.12720265 | 2 | 0.11 | 0.40 |
B.dentium | 2.636367 | 58.53672876 | 1 | 0.17 | 0.48 |
B.duttonii | 1.574881 | 28.01157675 | 1 | 0.08 | 0.43 |
B.longum | 3.614992 | 72.04577493 | 2 | 0.18 | 0.48 |
B.aphidicola_Cc | 0.705557 | 27.38318803 | 1 | 0.12 | 0.62 |
B.fragilis | 5.31099 | 43.20429901 | 2 | 0.19 | 0.55 |
B.fragilis_NCTC9343 | 5.2417 | 43.11418051 | 2 | 0.20 | 0.58 |
B.garinii | 1.230533 | 28.16974433 | 1 | 0.07 | 0.47 |
Burkholderia_383 | 7.884858 | 63.26677031 | 3 | 0.24 | 0.56 |
Burkholderia_CCGE1002 | 7.043595 | 63.24567213 | 3 | 0.24 | 0.60 |
B.cenocepacia | 7.284683 | 67.92633255 | 3 | 0.27 | 0.59 |
B.indica | 2.36952 | 38.03732401 | 1 | 0.18 | 0.49 |
B.licheniformis_DSM13 | 4.202352 | 43.6855123 | 1 | 0.18 | 0.44 |
B.japonicum | 1.931047 | 38.23034861 | 1 | 0.18 | 0.51 |
B.hermsii | 0.922307 | 29.82770379 | 1 | 0.11 | 0.54 |
B.recurrentis | 3.036634 | 27.00071856 | 1 | 0.05 | 0.38 |
Bradyrhizobium_BTAi1 | 4.418616 | 56.98295575 | 2 | 0.22 | 0.57 |
Bradyrhizobium_ORS278 | 9.105828 | 64.05938043 | 7 | 0.23 | 0.58 |
B.adolescentis | 1.933695 | 60.49035655 | 1 | 0.20 | 0.49 |
B.longum_longum_JDM301 | 2.265943 | 59.94603571 | 1 | 0.15 | 0.47 |
B.animalis_lactis | 1.938709 | 60.48213012 | 1 | 0.19 | 0.49 |
B.megaterium_QM_B1551 | 4.222645 | 46.19474287 | 1 | 0.16 | 0.44 |
B.megaterium_DSM319 | 4.222597 | 46.19439175 | 1 | 0.16 | 0.43 |
B.longum_infantis_ATCC15697 | 2.389526 | 60.16389861 | 1 | 0.15 | 0.45 |
B.faecium | 2.477838 | 59.81488701 | 1 | 0.18 | 0.51 |
B.longum_longum_BBMN68 | 2.832748 | 59.86314349 | 1 | 0.16 | 0.45 |
B.longum_DJO10A | 2.260266 | 60.12734784 | 1 | 0.16 | 0.50 |
B.animalis_lactis_Bl-04 | 1.938483 | 60.48216569 | 1 | 0.19 | 0.49 |
B.cenocepacia_HI2424 | 5.835527 | 68.48551982 | 2 | 0.25 | 0.54 |
B.grahamii | 3.286445 | 57.21933579 | 1 | 0.19 | 0.51 |
B.henselae | 3.283936 | 57.22166936 | 1 | 0.20 | 0.55 |
B.pseudofirmus | 5.097447 | 38.13163727 | 1 | 0.12 | 0.40 |
B.subvibrioides | 3.294931 | 57.22244259 | 1 | 0.20 | 0.55 |
B.abortus | 3.278307 | 57.22185262 | 1 | 0.19 | 0.51 |
B.abortus_S19 | 3.311219 | 57.22122276 | 1 | 0.19 | 0.52 |
B.mallei_NCTC10229 | 7.00881 | 66.6863419 | 3 | 0.23 | 0.57 |
B.cenocepacia_MC0-3 | 5.742303 | 68.46732052 | 2 | 0.24 | 0.51 |
B.cepacia | 5.84838 | 68.48459744 | 1 | 0.23 | 0.50 |
B.pumilus | 5.523192 | 37.92643819 | 1 | 0.11 | 0.40 |
B.canis | 3.337369 | 57.25433717 | 1 | 0.19 | 0.52 |
B.microti | 3.315175 | 57.25130649 | 1 | 0.19 | 0.50 |
B.ovis | 3.324607 | 57.20522757 | 1 | 0.19 | 0.51 |
B.mallei | 7.008622 | 66.68609036 | 3 | 0.24 | 0.57 |
B.glumae | 5.232401 | 68.4037978 | 1 | 0.23 | 0.50 |
B.melitensis | 3.27559 | 57.19455121 | 1 | 0.20 | 0.54 |
B.mallei_NCTC10247 | 4.773551 | 68.09995326 | 2 | 0.27 | 0.64 |
B.selenitireducens | 4.404886 | 40.00847014 | 1 | 0.13 | 0.44 |
B.pseudomallei_1710b | 7.040403 | 68.28915902 | 2 | 0.22 | 0.47 |
B.mallei_SAVP1 | 4.086189 | 67.72058267 | 2 | 0.31 | 0.65 |
B.subtilis | 4.249248 | 39.86055886 | 2 | 0.13 | 0.37 |
B.multivorans_T | 8.676562 | 62.29240337 | 2 | 0.23 | 0.57 |
Blattabacterium_Periplaneta_americana | 0.640442 | 28.21223468 | 1 | 0.21 | 0.68 |
B.pseudomallei | 7.089249 | 68.25797768 | 2 | 0.22 | 0.46 |
B.pseudomallei_1106a | 7.308054 | 67.97815123 | 2 | 0.31 | 0.58 |
B.hyodysenteriae | 2.586443 | 27.89699986 | 1 | 0.06 | 0.40 |
B.pseudomallei_668 | 4.098576 | 67.70876031 | 2 | 0.21 | 0.47 |
B.phytofirmans | 7.247547 | 68.05872387 | 3 | 0.27 | 0.59 |
B.multivorans | 5.28795 | 65.48282416 | 2 | 0.25 | 0.60 |
B.subtilis_spizizenii | 3.704465 | 41.28690648 | 2 | 0.15 | 0.42 |
B.phymatum | 8.214658 | 62.28682923 | 1 | 0.23 | 0.57 |
B.melitensis_Abortus | 1.581384 | 38.79683872 | 1 | 0.21 | 0.59 |
C.crescentus | 7.456587 | 65.51038699 | 4 | 0.20 | 0.55 |
B.murdochii | 1.242163 | 27.52565485 | 1 | 0.08 | 0.47 |
B.pseudomallei_MSHR346 | 3.750138 | 60.70797021 | 1 | 0.22 | 0.46 |
B.turicatae | 3.241804 | 27.75143099 | 1 | 0.06 | 0.40 |
B.melitensis_ATCC23457 | 3.445263 | 68.42270097 | 1 | 0.15 | 0.49 |
B.thuringiensis | 3.592487 | 48.66996039 | 1 | 0.16 | 0.40 |
B.thuringiensis_BMB171 | 4.027676 | 43.89067045 | 2 | 0.15 | 0.41 |
B.thuringiensis_AlHakam | 4.215606 | 43.51440813 | 1 | 0.14 | 0.41 |
B.proteoclasticus | 5.643051 | 35.17646748 | 1 | 0.11 | 0.39 |
B.rhizoxinica | 6.723972 | 67.62812219 | 4 | 0.28 | 0.59 |
B.thetaiotaomicron | 6.293399 | 42.86028901 | 1 | 0.22 | 0.60 |
B.weihenstephanensis | 5.314794 | 35.36523711 | 1 | 0.11 | 0.40 |
B.brevis | 5.31303 | 35.43373555 | 1 | 0.12 | 0.44 |
B.suis | 2.642404 | 38.82222401 | 1 | 0.17 | 0.47 |
A.thermophilum | 3.384766 | 59.11413078 | 1 | 0.27 | 0.56 |
B.pilosicoli | 0.91733 | 29.11896482 | 1 | 0.12 | 0.53 |
B.aphidicola_Sg | 0.655725 | 26.36486332 | 1 | 0.11 | 0.59 |
B.xenovorans | 8.676277 | 66.27244612 | 4 | 0.23 | 0.58 |
B.thailandensis | 8.39107 | 65.73805248 | 3 | 0.23 | 0.54 |
This is a portion of the data; to view all the data, please download the file. |
Dataset 1.Application of SolubEcoli.pgc and GDP1.pgc classifiers.
Proteome wide prediction of GroEL obligatory protein fraction (fC3) and aggregation prone protein fraction (fAg) in 1132 eubacterial genomes with their genome size, GC content and GroEL copy number.Conclusions
Several machine learning (ML) classifiers have been developed to predict aggregation-prone or GroEL-dependent proteins, but very few of them used data sets generated and curated from multiple experimental studies. Our classifiers were based on curated data from multiple studies and performed well also against the false positive C3 homologs of Ureaplasma, showing accuracy and noise tolerance. According to previous theories, GC-poor organisms might have evolved through population bottlenecks. This allows mildly deleterious mutations to be fixed in the population with a high probability2,44. It has been hypothesized that the GC-poor genomes that accumulated a large number of deleterious mutations in the course of evolution, through population bottlenecks and hence harbour proteins that are aggregation-prone. Although overexpressions of chaperones are observed in GC-poor organisms that have reduced genomes, there are also other GC-poor organisms that lack GroEL. Our work provides strong evidence that the general stability of the proteome increases with the decrease in GC content of eubacterial genomes. Decrease in GC content restricts the amino acid space that the organism can sample, thereby compromising protein evolution. We hypothesise that, even with this limited amino acid space, GC-poor organisms are still abundant as growth is facilitated under conditions that compromise protein folding capacity. This antagonism between ability to evolve and folding advantage could be crucial in facilitating protein evolution in the presence of chaperones and other folding machineries45–48.
Our work suggests that organisms facing continuous proteostasis problems would tend to shift towards a more GC-poor genome. This is supported by findings of Xia et al.49 who have reported that the preponderance of GC to AT conversions during high temperature laboratory adaptation of Pasteurella multocida. Further in vitro evolution experiments will be required to demonstrate whether laboratory adaptation to low GC content may provide folding advantage.
Data availability
F1000Research: Dataset 1. Application of SolubEcoli.pgc and GDP1.pgc classifiers, 10.5256/f1000research.4307.d2962455.
ZENODO: Training data of protein classifier SolubEcoli.pgc and GDP1.pgc, doi: 10.5281/zenodo.1044256.
Author contributions
KC and DD designed the study. RDR carried out all the analysis. MB and VB participated in the design and discussion of the study. RDR, KC and DD prepared the manuscript and all authors agreed to the final content.
Competing interests
No competing interests were disclosed.
Grant information
RDR acknowledges HP and CSIR for funding the study. DD and KC acknowledge CSIR for funding through IGIB. KC acknowledges Wellcome Trust – DBT India alliance for funding and fellowship.
The funders had no role in study design, data collection and analysis, decision to publish, or preparation of the manuscript.
Faculty Opinions recommendedReferences
- 1.
Nishida H:
Evolution of genome base composition and genome size in bacteria.
Front Microbiol.
2012; 3: 420. PubMed Abstract
| Publisher Full Text
| Free Full Text
- 2.
McCutcheon JP, Moran NA:
Extreme genome reduction in symbiotic bacteria.
Nat Rev Microbiol.
2012; 10(1): 13–26. PubMed Abstract
| Publisher Full Text
- 3.
Guo FB, Lin H, Huang J:
A plot of G + C content against sequence length of 640 bacterial chromosomes shows the points are widely scattered in the upper triangular area.
Chromosome Res.
2009; 17(3): 359–364. PubMed Abstract
| Publisher Full Text
- 4.
Lightfield J, Fram NR, Ely B:
Across bacterial phyla, distantly-related genomes with similar genomic GC content have similar patterns of amino acid usage.
PLoS One.
2011; 6(3): e17677. PubMed Abstract
| Publisher Full Text
| Free Full Text
- 5.
van Ham RC, Kamerbeek J, Palacios C, et al.:
Reductive genome evolution in Buchnera aphidicola.
Proc Natl Acad Sci U S A.
2003; 100(2): 581–586. PubMed Abstract
| Publisher Full Text
| Free Full Text
- 6.
Bastolla U, Moya A, Viguera E, et al.:
Genomic determinants of protein folding thermodynamics in prokaryotic organisms.
J Mol Biol.
2004; 343(5): 1451–1466. PubMed Abstract
| Publisher Full Text
- 7.
Fares MA, Moya A, Barrio E:
GroEL and the maintenance of bacterial endosymbiosis.
Trends Genet.
2004; 20(9): 413–416. PubMed Abstract
| Publisher Full Text
- 8.
Fares MA, Ruiz-Gonzalez MX, Moya A, et al.:
Endosymbiotic bacteria: groEL buffers against deleterious mutations.
Nature.
2002; 417(6887): 398. PubMed Abstract
| Publisher Full Text
- 9.
Moran NA:
Accelerated evolution and Muller’s rachet in endosymbiotic bacteria.
Proc Natl Acad Sci U S A.
1996; 93(7): 2873–2878. PubMed Abstract
| Publisher Full Text
| Free Full Text
- 10.
Aksoy S:
Molecular analysis of the endosymbionts of tsetse flies: 16S rDNA locus and over-expression of a chaperonin.
Insect Mol Biol.
1995; 4(1): 23–29. PubMed Abstract
- 11.
Clark MA, Baumann L, Baumann P:
Sequence analysis of a 34.7–kb DNA segment from the genome of Buchnera aphidicola (endosymbiont of aphids) containing groEL, dnaA, the atp operon, gidA, and rho.
Curr Microbiol.
1998; 36(3): 158–163. PubMed Abstract
| Publisher Full Text
- 12.
Wilcox JL, Dunbar HE, Wolfinger RD, et al.:
Consequences of reductive evolution for gene expression in an obligate endosymbiont.
Mol Microbiol.
2003; 48(6): 1491–1500. PubMed Abstract
| Publisher Full Text
- 13.
Williams TA, Fares MA:
The effect of chaperonin buffering on protein evolution.
Genome Biol Evol.
2010; 2: 609–619. PubMed Abstract
| Publisher Full Text
| Free Full Text
- 14.
Kerner MJ, Naylor DJ, Ishihama Y, et al.:
Proteome-wide analysis of chaperonin-dependent protein folding in Escherichia coli.
Cell.
2005; 122(2): 209–220. PubMed Abstract
| Publisher Full Text
- 15.
Chapman E, Farr GW, Usaite R, et al.:
Global aggregation of newly translated proteins in an Escherichia coli strain deficient of the chaperonin GroEL.
Proc Natl Acad Sci U S A.
2006; 103(43): 15800–15805. PubMed Abstract
| Publisher Full Text
| Free Full Text
- 16.
Raineri E, Ribeca P, Serrano L, et al.:
A more precise characterization of chaperonin substrates.
Bioinformatics.
2010; 26(14): 1685–1689. PubMed Abstract
| Publisher Full Text
- 17.
Bogumil D, Dagan T:
Chaperonin-dependent accelerated substitution rates in prokaryotes.
Genome Biol Evol.
2010; 2: 602–608. PubMed Abstract
| Publisher Full Text
| Free Full Text
- 18.
Niwa T, Kanamori T, Ueda T, et al.:
Global analysis of chaperone effects using a reconstituted cell-free translation system.
Proc Natl Acad Sci U S A.
2012; 109(23): 8937–8942. PubMed Abstract
| Publisher Full Text
| Free Full Text
- 19.
Calloni G, Chen T, Schermann SM, et al.:
DnaK Functions as a Central Hub in the E. coli Chaperone Network.
Cell Rep.
2012; 1(3): 251–264. PubMed Abstract
| Publisher Full Text
- 20.
Tartaglia GG, Dobson CM, Hartl FU, et al.:
Physicochemical determinants of chaperone requirements.
J Mol Biol.
2010; 400(3): 579–588. PubMed Abstract
| Publisher Full Text
- 21.
Noivirt-Brik O, Unger R, Horovitz A:
Low folding propensity and high translation efficiency distinguish in vivo substrates of GroEL from other Escherichia coli proteins.
Bioinformatics.
2007; 23(24): 3276–3279. PubMed Abstract
| Publisher Full Text
- 22.
Fang Y, Fang J:
Discrimination of soluble and aggregation-prone proteins based on sequence information.
Mol BioSyst.
2013; 9(4): 806–811. PubMed Abstract
| Publisher Full Text
| Free Full Text
- 23.
Stiglic G, Kocbek S, Pernek I, et al.:
Comprehensive decision tree models in bioinformatics.
PLoS One.
2012; 7(3): e33812. PubMed Abstract
| Publisher Full Text
| Free Full Text
- 24.
Klus P, Bolognesi B, Agostini F, et al.:
The cleverSuite Approach for Protein Characterization: Predictions of Structural Properties, Solubility, Chaperone Requirements and RNA-Binding Abilities.
Bioinformatics.
2014; 30(11): 1601–1608. PubMed Abstract
| Publisher Full Text
| Free Full Text
- 25.
Niwa T, Ying BW, Saito K, et al.:
Bimodal protein solubility distribution revealed by an aggregation analysis of the entire ensemble of Escherichia coli proteins.
Proc Natl Acad Sci U S A.
2009; 106(11): 4201–4206. PubMed Abstract
| Publisher Full Text
| Free Full Text
- 26.
Fujiwara K, Ishihama Y, Nakahigashi K, et al.:
A systematic survey of in vivo obligate chaperonin-dependent substrates.
EMBO J.
2010; 29(9): 1552–1564. PubMed Abstract
| Publisher Full Text
| Free Full Text
- 27.
Li W, Godzik A:
Cd-hit: a fast program for clustering and comparing large sets of protein or nucleotide sequences.
Bioinformatics.
2006; 22(13): 1658–1659. PubMed Abstract
| Publisher Full Text
- 28.
Das Roy R, Dash D:
Selection of relevant features from amino acids enables development of robust classifiers.
Amino Acids.
2014; 46(5): 1343–1351. PubMed Abstract
| Publisher Full Text
- 29.
Zweig MH, Campbell G:
Receiver-operating characteristic (ROC) plots: a fundamental evaluation tool in clinical medicine.
Clin Chem.
1993; 39(4): 561–577. PubMed Abstract
- 30.
Uchiyama I, Higuchi T, Kawai M:
MBGD update 2010: toward a comprehensive resource for exploring microbial genome diversity.
Nucleic Acids Res.
2010; 38(Database issue): D361–D365. PubMed Abstract
| Publisher Full Text
| Free Full Text
- 31.
Hill JE, Penny SL, Crowell KG, et al.:
cpnDB: a chaperonin sequence database.
Genome Res.
2004; 14(8): 1669–1675. PubMed Abstract
| Publisher Full Text
| Free Full Text
- 32.
Pruesse E, Quast C, Knittel K, et al.:
SILVA: a comprehensive online resource for quality checked and aligned ribosomal RNA sequence data compatible with ARB.
Nucleic Acids Res.
2007; 35(21): 7188–7196. PubMed Abstract
| Publisher Full Text
| Free Full Text
- 33.
Team RC: R: A language and environment for statistical computing. R foundation for Statistical Computing. 2005. Reference Source
- 34.
Midford P, Garland T Jr, Maddison W:
PDAP Package of Mesquite. Version 1.14. 2008. Reference Source
- 35.
Mesquite: a modular system for evolutionary analysis. Version 2.75. Reference Source
- 36.
Vapnik V:
The nature of statistical learning theory. 2nd edition. Springer; 1999. Reference Source
- 37.
Chou KC:
Prediction of protein cellular attributes using pseudo-amino acid composition.
Proteins.
2001; 43(3): 246–255. PubMed Abstract
| Publisher Full Text
- 38.
Prilusky J, Felder CE, Zeev-Ben-Mordehai T, et al.:
FoldIndex©: a simple tool to predict whether a given protein sequence is intrinsically unfolded.
Bioinformatics.
2005; 21(16): 3435–3438. PubMed Abstract
| Publisher Full Text
- 39.
Singh GP, Dash D:
Electrostatic mis-interactions cause overexpression toxicity of proteins in E. coli.
PLoS One.
2013; 8(5): e64893. PubMed Abstract
| Publisher Full Text
| Free Full Text
- 40.
Bohlin J, Brynildsrud O, Vesth T, et al.:
Amino acid usage Is asymmetrically biased in AT-and GC-rich microbial genomes.
PLoS One.
2013; 8(7): e69878. PubMed Abstract
| Publisher Full Text
| Free Full Text
- 41.
Garland T, Bennett AF, Rezende EL:
Phylogenetic approaches in comparative physiology.
J Exp Biol.
2005; 208(Pt 16): 3015–3035. PubMed Abstract
| Publisher Full Text
- 42.
Mazurie A, Bonchev D, Schwikowski B, et al.:
Evolution of metabolic network organization.
BMC Syst Biol.
2010; 4: 59. PubMed Abstract
| Publisher Full Text
| Free Full Text
- 43.
Clark GW, Tillier ER:
Loss and gain of GroEL in the Mollicutes.
Biochem Cell Biol.
2010; 88(2): 185–194. PubMed Abstract
| Publisher Full Text
- 44.
Mira A, Moran NA:
Estimating population size and transmission bottlenecks in maternally transmitted endosymbiotic bacteria.
Microb Ecol.
2002; 44(2): 137–143. PubMed Abstract
| Publisher Full Text
- 45.
Bandyopadhyay A, Saxena K, Kasturia N, et al.:
Chemical chaperones assist intracellular folding to buffer mutational variations.
Nat Chem Biol.
2012; 8(3): 238–245. PubMed Abstract
| Publisher Full Text
| Free Full Text
- 46.
Rutherford SL, Lindquist S:
Hsp90 as a capacitor for morphological evolution.
Nature.
1998; 396(6709): 336–342. PubMed Abstract
| Publisher Full Text
- 47.
Queitsch C, Sangster TA, Lindquist S:
Hsp90 as a capacitor of phenotypic variation.
Nature.
2002; 417(6889): 618–624. PubMed Abstract
| Publisher Full Text
- 48.
Rohner N, Jarosz DF, Kowalko JE, et al.:
Cryptic variation in morphological evolution: HSP90 as a capacitor for loss of eyes in cavefish.
Science.
2013; 342(6164): 1372–1375. PubMed Abstract
| Publisher Full Text
| Free Full Text
- 49.
Xia X, Wei T, Xie Z, et al.:
Genomic changes in nucleotide and dinucleotide frequencies in Pasteurella multocida cultured under high temperature.
Genetics.
2002; 161(4): 1385–1394. PubMed Abstract
- 50.
Chou KC:
Prediction of protein subcellular locations by incorporating quasi-sequence-order effect.
Biochem Biophys Res Commun.
2000; 278(2): 477–483. PubMed Abstract
| Publisher Full Text
- 51.
Bum Ju L, Keun Ho R:
Feature Extraction from Protein Sequences and Classification of Enzyme Function. In BioMedical Engineering and Informatics, 2008 BMEI 2008 International Conference on; 27–30 May 2008. 2008; 138–142. Publisher Full Text
- 52.
Shamim MT, Anwaruddin M, Nagarajaram HA:
Support Vector Machine-based classification of protein folds using the structural properties of amino acid residues and amino acid residue pairs.
Bioinformatics.
2007; 23(24): 3320–3327. PubMed Abstract
| Publisher Full Text
- 53.
Li ZR, Lin HH, Han LY, et al.:
PROFEAT: a web server for computing structural and physicochemical features of proteins and peptides from amino acid sequence.
Nucleic Acids Res.
2006; 34(Web Server issue): W32–W37. PubMed Abstract
| Publisher Full Text
| Free Full Text
- 54.
Dubchak I, Muchnik I, Holbrook SR, et al.:
Prediction of protein folding class using global description of amino acid sequence.
Proc Natl Acad Sci U S A.
1995; 92(19): 8700–8704. PubMed Abstract
| Free Full Text
- 55.
Das Roy R, Bhardwaj M, Bhatnagar V, et al.:
Application of SolubEcoli.pgc and GDP1.pgc classifiers.
F1000Research.
Data Source
- 56.
Das Roy R, Chakraborty K, Das D:
Training data of protein classifier SolubEcoli.pgc and GDP1.pgc. 2014. Data Source
Comments on this article Comments (0)